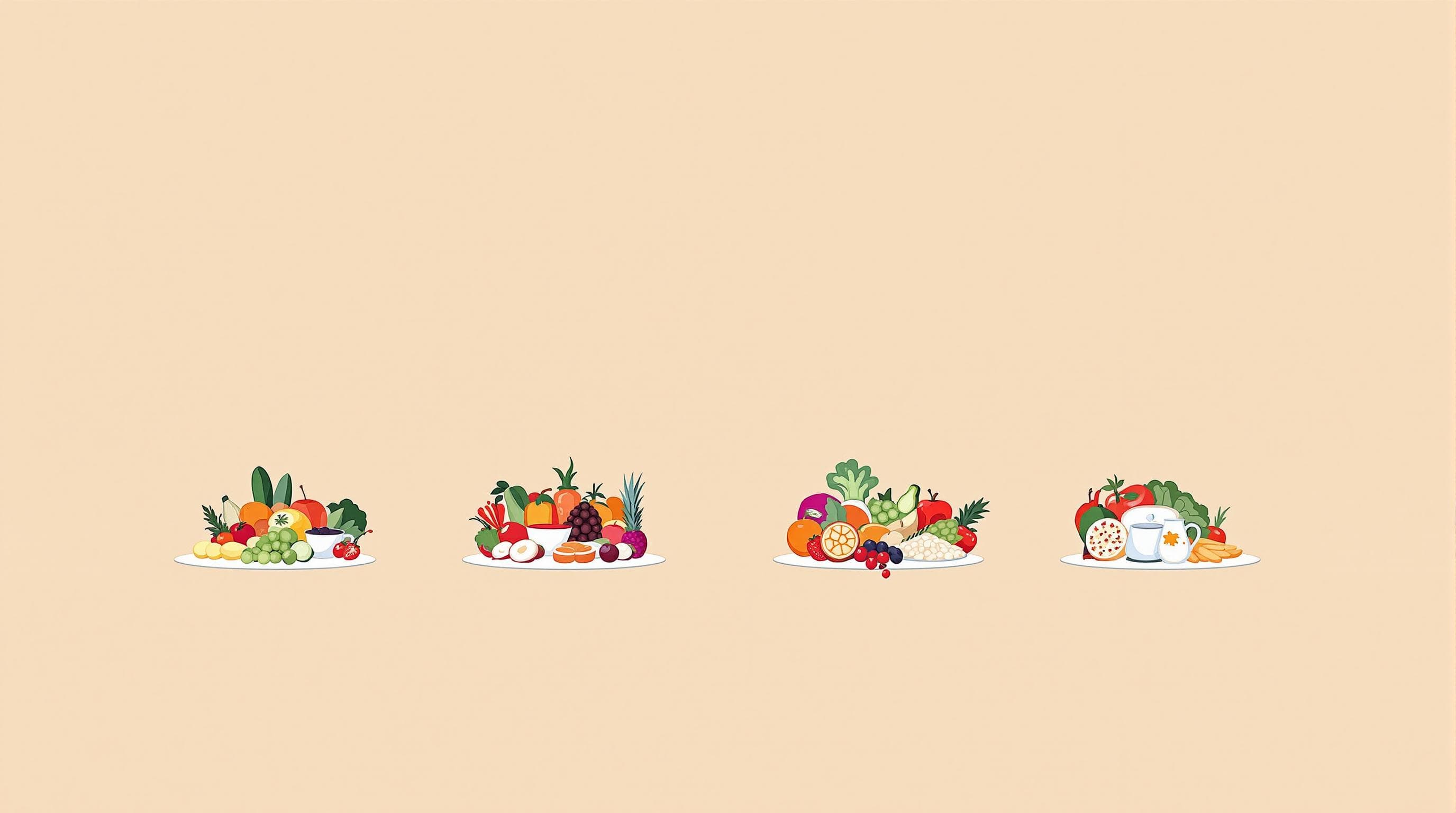
AI in Nutrition: Clustering for Personalized Diets
AI is transforming how we approach nutrition by enabling personalized diets through clustering techniques. Here's what you need to know:
- Why Personal Diets? Tailored plans improve adherence and results by considering metabolism, genetics, and lifestyle.
- AI's Role: Clustering methods like K-means, hierarchical, and density-based algorithms analyze eating habits, meal timing, and nutrient intake to create custom recommendations.
- Key Applications:
- K-Means: Groups people by dietary profiles.
- Hierarchical Clustering: Maps nutrient relationships.
- Density Clustering: Handles complex, unstructured data.
- Practical Use: Nutritionists can design meal plans for athletes, weight management, or specific health conditions.
- Tools Like Supps AI: Platforms analyze data to refine supplement and diet choices while ensuring data privacy.
AI-powered clustering is reshaping nutrition by turning complex data into actionable, personalized diets. However, ethical concerns like data privacy and algorithmic fairness must be addressed to ensure trust and transparency.
Towards personalized diet using linked data
Types of Clustering in Diet Analysis
AI-driven clustering techniques analyze extensive dietary data to uncover patterns and group individuals or behaviors. Here’s a closer look at methods like K-means, hierarchical, and density-based clustering, which help identify dietary trends.
K-Means for Diet Groups
K-means clustering is a go-to method for grouping individuals based on eating habits and nutritional intake. The algorithm refines clusters through repeated iterations until it identifies the best groupings.
Feature | Role in Diet Analysis |
---|---|
Data Points | Evaluates caloric intake, macronutrient ratios, and meal timing |
Centroids | Defines typical dietary profiles |
Distance Metrics | Assesses similarities in eating behaviors |
Iterations | Adjusts groupings for optimal results |
This method helps categorize people into clear dietary profiles, making it easier to tailor nutrition advice.
Hierarchical Analysis of Nutrients
Hierarchical clustering maps relationships between nutrients and their effects on health. By building a branching diagram, it highlights connections among dietary components. This method is particularly helpful for:
- Pinpointing nutrient combinations that work well together
- Linking supplement intake to overall diet quality
- Understanding how various food groups contribute to nutrition
These insights enable more precise, nutrient-focused dietary recommendations.
Density Clustering for Diet Data
Density-based clustering, such as DBSCAN, is effective for analyzing messy or unstructured dietary data. It forms clusters based on proximity, making it ideal for uncovering natural groupings in complex datasets.
Application | Insight Provided |
---|---|
Meal Pattern Analysis | Identifies common eating schedules and preferences |
Supplement Interactions | Highlights frequent supplement combinations |
Food Choice Patterns | Shows natural groupings in food selection |
Outlier Detection | Flags unusual or concerning eating behaviors |
This approach works well for datasets that don’t fit traditional categories, helping to reveal patterns that support personalized nutrition strategies as digital tracking tools grow in popularity.
Using Clusters for Diet Planning
Group-Based Diet Analysis
Clustering transforms dietary data into actionable group insights. These algorithms study food choices, meal timing, and nutrient intake to generate focused recommendations. Nutritionists can use these clusters to identify common eating habits and design interventions tailored to specific groups.
Population Group | Benefits of Clustering | Practical Use |
---|---|---|
Athletes | Highlights ideal nutrient timing | Pre- and post-workout meal plans |
Weight Management | Pinpoints effective eating habits | Long-term diet strategies |
Health Conditions | Groups shared dietary requirements | Customized meal plans |
Age Groups | Identifies generational trends | Age-specific portion guidelines |
Meal Planning with Clusters
Cluster analysis enables the creation of personalized meal plans by uncovering successful eating patterns. Instead of generic advice, this method focuses on solutions grounded in actual eating behaviors.
How It’s Used:
- Pinpointing the best meal times based on activity-based clusters.
- Adjusting portion sizes to match individual metabolic needs.
- Designing meal options that align with specific food preference clusters.
Supps AI and Clustering
Platforms like Supps AI take these insights further, applying clustering to refine supplement recommendations. By analyzing user data, Supps AI delivers tailored guidance while ensuring data privacy through encryption.
"Love how it tracks interactions between different supplements. The detailed analysis of each ingredient has helped me optimize my supplement stack." - Emma R.
Supps AI supports more than 1,000 users and offers features such as:
- Barcode scanning for instant supplement evaluations.
- Health score tracking based on clustered patterns.
- Ingredient interaction monitoring to avoid conflicts.
- Efficacy and risk assessments for smarter supplement choices.
With its clustering capabilities, the platform helps users make informed decisions about supplements that match their unique health needs and dietary habits. This data-driven approach combines scientific accuracy with practical, easy-to-follow recommendations.
sbb-itb-79d218a
Limits and Ethics
Data and Privacy Issues
AI uses dietary data to create personalized nutrition plans, but keeping this data secure is crucial. Here are some key privacy measures:
- Data encryption: Personal health information must be safeguarded with industry-standard security protocols.
- User consent: Users should explicitly opt in before their data is collected.
- Data retention: Personal health information should only be stored for a defined period.
- Access control: Strict rules must govern who can access and analyze user data.
Making Sense of Clusters
Turning AI clustering results into practical dietary advice isn't always straightforward. Variations among individuals and inconsistencies in data require careful analysis and validation. These technical hurdles often raise ethical concerns about fairness and accountability in recommendations.
Ethics of AI Diet Advice
AI-driven dietary advice introduces ethical challenges, particularly around algorithmic bias, which could lead to unfair or inappropriate recommendations.
Key ethical practices include:
- Clearly explaining how recommendation algorithms work.
- Ensuring human oversight remains part of the process.
- Regularly reviewing algorithms to identify and address potential biases.
- Transparently communicating the limitations of AI-generated advice.
Platforms using AI for diet recommendations should explain how their systems work, include disclaimers about what AI can and can't do, and ensure humans are involved in the decision-making process. Algorithms should be updated regularly based on the latest research and user feedback. Balancing technological advances with strong data protection and ethical standards is essential to keep AI-driven dietary advice safe, transparent, and trustworthy.
What's Next for AI Diet Planning
Wearables and Diet Data
AI-powered wearables are reshaping how we approach personalized nutrition. Devices like smartwatches, fitness trackers, and continuous glucose monitors now provide real-time insights into how our bodies respond to food. These gadgets monitor key health metrics, offering a deeper understanding of individual metabolic patterns. For instance, Supps AI links personal health data to its supplement recommendation system, delivering tailored suggestions based on your unique needs. This constant flow of information paves the way for more advanced analysis methods in the future.
Advanced Clustering Techniques
With richer data inputs, next-gen algorithms are set to go beyond the basics. These systems will analyze a wide range of factors, including genetic traits, microbiome health, environmental influences, lifestyle habits, and supplement effects. Instead of relying on broad demographic categories, these algorithms will dive into multi-layered data, identifying subtle patterns in how individuals respond to different diets.
Collaboration Between AI Tools
As AI tools grow more sophisticated, combining their capabilities becomes crucial. A great example is Supps AI, which merges supplement recommendations with personalized health tracking. One user shared her experience:
"Supps AI has completely changed how I choose my supplements. It's like having a nutritionist in my pocket!" – Sarah J.
Conclusion
AI's Role in Nutrition
AI-driven clustering methods are reshaping how we approach personalized nutrition. By decoding complex patterns in eating habits and nutritional requirements, these tools provide tailored recommendations far beyond the scope of one-size-fits-all diets. The combination of machine learning and nutritional science has made it possible to craft diets that align closely with individual health objectives.
Ethics and Challenges
Maintaining a balance between innovation and protecting user data is crucial. These ethical considerations highlight the need for platforms that combine thorough analysis with a user-first approach.
Supps AI's Impact
Supps AI showcases how AI-powered clustering can turn data into meaningful dietary guidance. The platform stands out for its ability to simplify decision-making with smart clustering and in-depth analysis. As David K. puts it:
"The transparency in supplement ratings is incredible. No more guessing about quality or effectiveness. This app has become my go-to supplement advisor".